Hi all,
Happy new year! My first post this year will be about an idea that I had with my friend Ricardo Caspirro about the next generation of social recommenders in commerce applications and retail stores. What excites me is that this idea came from a conversation that we had in 2009, and since that year we discussed more about what it would be the "Deepjewel".
Deepjewel is a giant knowledge base that encapsulates interesting entities and relationships of the social world in the web. The social world in this context means all the millions and billions of tweets, Facebook messages, profiles, relationships, blog posting, YouTube videos, and more - a living organism itself, constantly evolving.
 |
The Deepjewel |
But what motivated us to create the Deepjewel? One of main problems that we face nowadays is the discovery of content and items of our interest. Many times, for instance, to find a book or a movie that we like, it is required to search at several websites and social networks through the web. There isn't a tool that allow this connection between items of many domains in a organized and structured way, even for easy access. Those objects are spread over the web, and the recommendations are placed in social networks by comments, results of machine learning techniques or by queries at several web pages or search engines. The problem becomes worst when we don't know anything about the existence of a certain item, which it could result at never finding out that possible item that would be of our interest.
The social media is huge and we need tools that performs a deep analysis of all this data, filter out items of my interest, specially from the historical data (with our permission, of course) from our presence in the web and bring items and products relevant to us without loosing the discovery process associated with the serendipity. One of the solutions is a powerful recommender engine fed by this Social Genome.
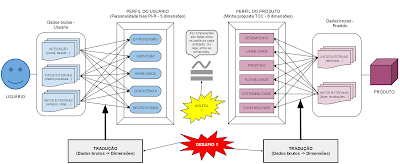 |
Hybrid Social Context-Aware Recommender RecDay |
This hybrid social context-aware recommender (which we call recday) is a engine composed by several modular components, which we employ a broad range of semantic analysis techniques, including information extraction and integration, natural language processing and machine learning. The main task of this recommender is to analyze information about his posts, bios and relationships/lists collected from the social genome and summarize it (all this data would represent the interests of the user) in profiles, which we could call DNA. Those profiles built by the recday would infer the possible interests of the users and would serve as basis for personalized recommendations of products and services from the retail stores/e-commerce applications.
A perfect example for this proccess, which we call the
translation, is when you mentioned several times about Apple products (such as macbook, ipod, iphone, etc) at your tweets. Even you never used the word "Apple", we can use the Social Genome to detect the products and infer that you are interested in Apple products. The following figure illustrate certain kinds of entities and relationships collected in the Social Genome:
The relationships extracted from the social web data
The second step of this engine is to build the user profile. Different from another approaches which it only uses the content or the historical data from reviews or ratings from the user, the Recday would go further and would analyze the temporal context included in the interests of the user. Several reports on consumer behavior show that the user desires are influented by external factors and even the humour or feelings of the person at the certain moment. It is required to collect in a stealth way (with the user permission of course) his social data and build his personality defined by several dimensions. Those dimensions represent the current state of the user which may define what kind of suggestions he would like to receive at that particular moment. For example, if you are happy today because you got a new job and posted at your Facebook about that event or even updated your profile about this new position, it would be a valuable information for your DNA profile in order to recommend products and services to celebrate this occasion (You are happy and excited, don't you think ?).
Another important component in this proccess is the product side. We need extract more information from their products portfolio. Items must be juiced in order to get all its meta-data available. Imagine the movie Batman where we have details about the year, genre, cast, production, direction, sinoypsis, etc. All this data can be used to build the DNA Item and be expressed by a collection of dimensions that represent the item profile. With those profiles (DNA User and DNA Item) we compute the similarity between user and items in order to produce a list of top recommendations and related explanations.
 |
The Social Architecure of the Recommender |
The final result can be shown in several mediums: mobile apps, widgets, web, API's, pluggins, etc. It is important the usability and how you will present all this information for a particular user. That's why the user interface must be simple and easy to navigate and have mechanisms to collect the user's feedback for the suggestions given by the engine. This proccess is cyclic, so when you give a feedback (a like or dislike or a comment about the suggestion), this piece of information is handled and leveraged to power your DNA profile.
 |
A particular medium of the recommendations: Ipad Demo |
In order to build all these interesting technical challenges, we needed to start developing our in-house solution called Crab, which proccesses all this data and employ several analysis and filtering techniques to deal with the percularities of this heterogeneous sources of data. The first start is the launch of the Deepjewel Labs. Deepjewel is a principle that we can mine the wealth in data, by identifying interesting entities and relationships and converting them into valuable information as input in the recommendation proccess of items and services.
In summary, all those human and computation techniques can be used to perform a deep semantic analysis of web and social data, where the result for a commerce application or retail store is the ability to offer what the users want before they know that they really want in a personalized way. The RecDay would be able to daily offer relevant product and services to their customers without they even know it exists. This is a new way to shop in which you don't have to go find products, service and information; the machine will help them find their way to you.
To know more about our Deepjewel labs, visit the website (currently in portuguese):
I hope you enjoyed,
Marcel Caraciolo